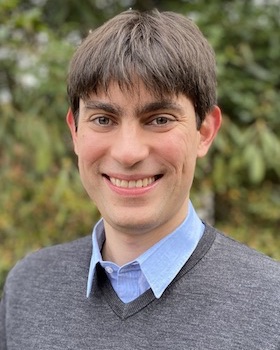
Pablo Videla
Understanding molecule-interface interactions: SFG of proteins, catalysts and hydrocarbonsUnderstanding the interaction of molecules with interfaces is of fundamental importance since a lot of processes are controlled by the particular way the molecule and surface ‘talk’ to each other. For example, the catalytic efficiency for the reduction of CO2 to CO by surface-immobilized Rhenium complexes crucially depends on the particular crystal face of the substrate used, whereas the stability of molecules of environmental importance, such as terpenes or carbamates, varies depending on particular surface-molecule interactions. One of the main focus of the group is to unravel how the underlying substrate-adsorbate interactions modulated the orientation, energetics and reactivity of different molecules at interfaces using a variety of computational methods ranging from Molecular Dynamics simulations to Quantum Mechanics calculations. Since a lot of the experimental information of substrate-molecule interactions come from surface-specific spectroscopies such as Sum Frequency Generation (SFG), the development of theoretical models to simulate and interpret the different spectral features of the SFG spectra is an active research area of the group. By combining electronic structure calculations with computational modelling, we have simulated both 1D-SFG and 2D-SFG for rigid and flexible molecules on different interface environment, providing insight into the interpretation of congested experimental spectral signals and disentangling the influence of surface interactions in determining molecular structure, orientation and ordering at the interfaces. This project involves an active collaboration with different experimental groups: Elsa Yan, Clifford Kubiak, Tianquan Lian, Poul Petersen, Luis Vellarde, and Franz Geiger.
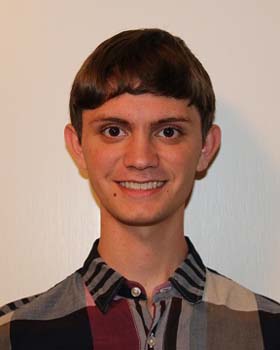
Ray Kelly
Controlling Catalysis with Electric FieldsWe are designing catalytic systems that allow for the control of reaction thermodynamics and kinetics by electric fields. The attachment of molecular catalysts to electrode surfaces enables their control by interfacial electric fields. Our fundamental theoretical studies provide design rules for catalysts that can exploit electric field effects. Simply modulating the applied potential can significantly alter the reactivity of surface-attached catalysts without any chemical modification.
We have theoretically demonstrated the precise control of the hydricity of a surface-attached catalyst, and have shown a field-switchable oxidative addition/reductive elimination reaction for a Vaska’s complex analogue. The dependence of these reactions on the metal center and substrate have been investigated to obtain design rules for improved catalytic systems. We are especially interested in understanding the inductive effect of an applied electric field on the metal center of surface-attached catalysts. Spectroelectrochemical simulations and experiments can help us understand to characterize the inductive effect so that we can understand how to harness it in catalytic systems. The insights from these studies are being used to design field-controllable catalytic systems with unprecedented reactivity. This work is done in collaboration with the experimental groups of Tianquan Lian at Emory University and Clifford Kubiak at the University of California, San Diego.
Catalyst Design and Mechanisms
Addressing contemporary challenges in alternative energy requires the design of improved electrocatalysts with higher selectivity and selectivity. As part of the Yale Solar Group Collaboration (with Brudvig and Crabtree),we are working on new electrocatalysts for water and ammonia oxidation using earth-abundant metals. In particular, we have studied the effect of changing the ligand and metal center on catalytic activity with a focus on how these changes affect coupled proton and electron transfer steps.
Additionally, we are working to understand catalysis on noble metal surfaces. We previously demonstrated that cis-selective transfer hydrogenation from tertiary amines to alkynes is enabled by electronic stabilization of a key surface-bound intermediate at high coverage. Currently, we are investigating the aromatization of cyclic enones to form phenols. DFT calculations elucidate the effects of metal surface on reactivity, showing that the heterogenous mechanism involves stabilization of the enol tautomer by the surface. Changing the metal alters the binding energies of the intermediates and the H donor and acceptor properties of the surface to control reactivity. This work is a collaboration with the experimental groups of Eszter Baráth at Leibniz Institute for Catalysis and Gary Haller at Yale.
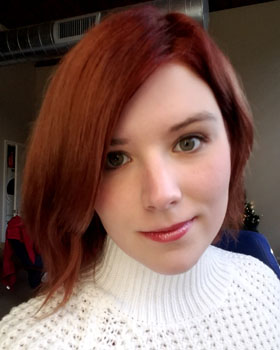
Jessica Freeze
Machine LearningInverse design of molecules for applications in green energy and environmental resuscitation can be greatly aided by implementation and design of machine learning methods. Predicting properties of catalytic interest from cheaply provided input parameters offers increased throughput and speed in the generation of crucial innovations for preserving and healing our environments. Additionally, the utilization of machine learning to determine chemically understandable functions offers the ability to break the scaling paradigms of current methods, as well as provide oracles for quantum computing applications.
Ammonia Oxidation
Destruction of waterways through nitrification from fertilizer run-off as well as the need to provide safe, green energy options present current and pressing problems. Designing catalysts to oxidize ammonia to nitrogen gas may be a solution. Efficient design of these catalysts and understanding their mechanisms will assist in these goals. Throughout design it is paramount that implementation side effects be examined and optimized so as to avoid possible harm via their insertion into the environment.
Quantum Computing
The promise of exponential speedup and the inclusion of interactions previously thought to be too expensive in chemical calculations positions quantum computing as a key asset for future chemists. In the regime of near-term quantum computers, we aim to guide innovation towards solving real chemical challenges on the quantum computers of today.
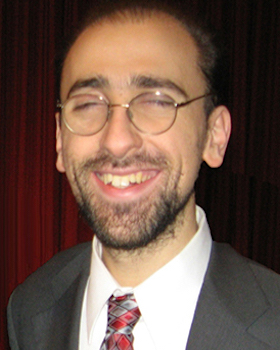
Matthew Guberman-Pfeffer
From the modeling of antigen recognition to the spectral modulation of light harvesting pigments and the structural basis for electrically conductive bacterial filaments, my research seeks to disclose the “secret motions and causes” of phenomena “for the effecting of all things possible” (Sir Francis Bacon), I love using computational methods to obtain structural or mechanistic insights that may aid the development of new materials. My postdoctoral research currently focuses on elucidating the mechanisms of energy and electron transfer in natural and synthetic systems.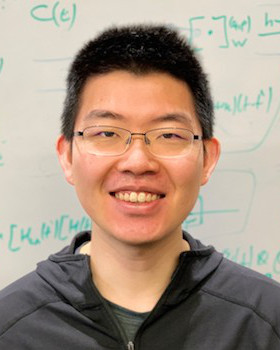
Ningyi Lyu
Quantum Dynamics with Tensor Decomposition MethodsRecent developments in tensor decomposition methods have helped to alleviate the exponentially high burden for computations on high-dimensional tensors. Since the many-body quantum system wavefunctions are by postulation high-dimensional tensors, these methods provide practical tools to compute, for example time propagation, on these wavefunctions. My research focuses on exploiting tensor decomposition methods to simulate molecular properties that are of chemical interest.
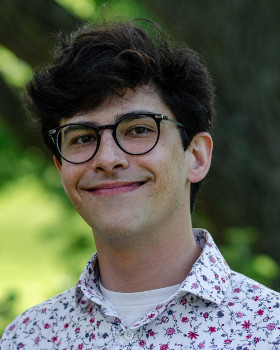
Brandon Allen
My research is mainly focused on understanding the biophysical mechanisms behind protein conformational changes through molecular dynamics and quantum dynamics. These dynamics are analyzed with tools employed from graph theory, information theory, and network analysis. I am also interested in quantum computers, specifically in their application to understanding biological systems.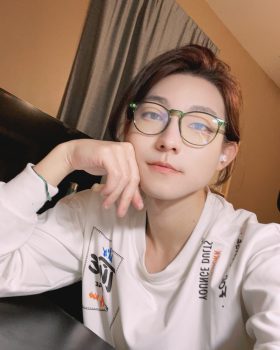
Haote Li
Machine learning is a rapidly evolving field that brings new ideas into academia every day. My research focuses on combining machine learning with chemistry. The goal is to develop novel methods that aid new chemistry findings. For example, my past works involve using the sequence-to-sequence model to generate new molecules with desired chemical properties and predicting time-evolving functions that explain chemical phenomena. In addition, since the invention of the graphical neural network (GNN) has created a very natural description of molecules, I am studying this model to make accurate predictions for the NMR shifts of molecules and protein primary sequences.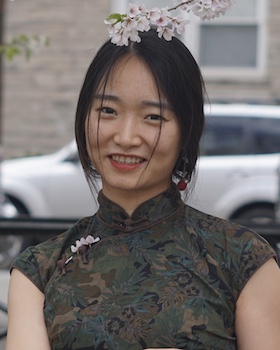
Jinchan Liu
Nature oxidizes water to dioxygen with the oxygen evolving complex (OEC) during photosynthesis in PSII, which serves as the blueprints for future clean energy technologies. The OEC is composed of a calcium, 5 µ-oxo oxygen and four high valence manganese, cooperating with a Yz· to catalyze the water oxidation reaction. My research focus on running calculations to provide insights on the mechanism of water oxidation. Currently, (1) I use quantum mechanics/molecular mechanics (QM/MM) to interrogate the water nucleophilic attack mechanism, especially how the cofactor Yz· is involved. (2) I am currently developing a force field for transition metal ions like Mn2+ to describe the interactions between water and the metal ions in molecular dynamics (MD). I will further apply this force field to the OEC to calculate the water exchange rate of water around the OEC, which will help to identify the substrate waters.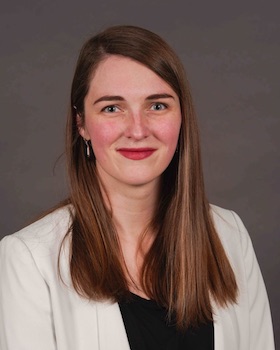
Ellen Mulvihill
Quantum Dynamics in Complex SystemsQuantum dynamics simulations are central to theoretical studies of many areas of chemistry and technology, including charge and energy transfer in photosynthetic and photovoltaic systems and a wide range of reactions with non-adiabatic dynamics and photochemical processes, including spin and vibrational energy relaxation. Despite considerable progress over the past few decades, the development of efficient methods for simulating quantum dynamics remains a challenge due to the computational cost of quantum-mechanically exact simulations, which scales exponentially with the number of degrees of freedom in the system.
Reduced-dimensionality approaches that can offer more favorable scaling provide a method for simulating quantum dynamics in complex systems. The generalized quantum master equation (GQME) is arguably the most general framework for simulating reduced dynamics, as it requires no approximations in its derivation. The GQME-based methodology allows one to restrict the dynamical input of the surroundings to short times via the memory kernel while calculating the long-time dynamics of the system of interest through the GQME.
Along with the GQME methodology, I am also exploring other methods for simulating quantum dynamics, including the time-dependent functional tensor-train Chebyshev (TD-FTTC) method. This TD-FTTC method extends the functionality of the FTTC method to be able to simulate time-dependent Hamiltonians, broadening the applicability of the FTTC method.
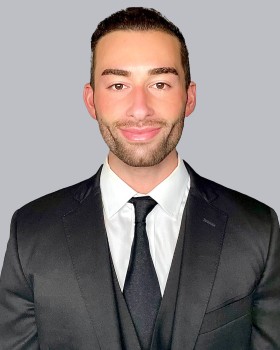
Gregory Kyro
Machine Learning for Predicting Biochemical PhenomenaMachine Learning, a subset of Artificial Intelligence, has proven to be a powerful tool for making predictions by discovering and interpreting patterns in data according to a set of algorithms. In particular, concepts from both graph theory (in the form of Graph Convolutional Networks, or GCNs) and image detection (in the form of Convolutional Neural Networks, or CNNs) are beneficial for extracting features from 3-dimensional protein structures. We have created an ensemble of attention-based convolutional neural networks, specifically a 3D-CNN and a message-passing GCN, for predicting protein-ligand binding affinity. We are applying this model to a variety of protein-ligand pairs to assist other projects. The primary goal is to generalize and extend this model to address biochemical questions that have not yet been formulated.
Statistical Methodologies for Studying Allostery in Biomolecules
The function of many biomolecular systems relies on transferring information between distant sites, a process known as allostery. Molecular Dynamics simulations allow us to analyze chemical phenomena that emerge over a timespan, such as the dynamics and motions that contribute to allostery. By representing a protein as a network, and calculating correlation of some quantity between each residue pair (i.e., atomic displacements, electrostatic energy, etc.), we are able to determine how central each residue is to the protein network under investigation, and therefore its contribution to the protein’s allosteric function. We have recently developed a new method based on this approach that is predicated on backbone electrostatics. One goal is to determine the allosteric mechanism of the gene-editing system CRISPR-Cas9 at a molecular resolution, which has not yet been established. We are also applying this approach to other popular systems such as IGPS and MIF.
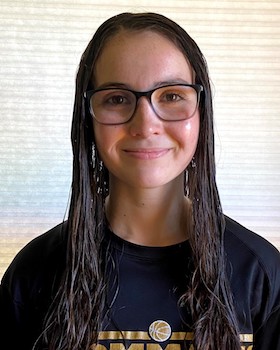
Abby Cuomo
Machine Learning in Organic SynthesisOrganic synthesis is extremely time and resource intensive as oftentimes reactions do not work as researchers planned they would. With the recent rise in the usage of machine learning, it is now possible to create models to aid scientists in the design of synthetic routes to accomplish their goals. As a member of the Batista and Newhouse labs, I use DFT and machine learning methods to predict yield, enantioselectivity and the structure of optimal substrates for reactions. I also use DFT to explain the selectivity or reversibility for the organic reactions that are run in the Newhouse lab.
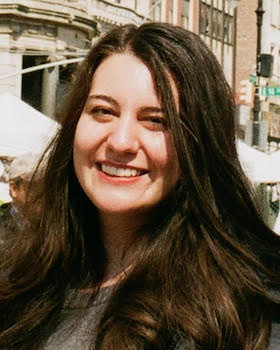
Jana Jelusic
Mechanistic Investigations: Ammonia Oxidation and Nitrate ReductionPhotoelectrochemical oxidation of ammonia is an attractive route to generating nitrites and nitrates which play a vital role in the production of fertilizers and feedstocks. In collaboration with the Brudvig Group, we are developing catalysts that can perform this reaction with low overpotential, high turnover frequency, and high stability. To develop a chemical understanding of such catalyst’s performance, analyses of the mechanisms of nitrite and nitrate formation are needed. We utilize quantum ab initio calculations of potential reaction pathways, and verify these results against experimentally obtained data.
Fertilizer runoff from agricultural activity leads to a release of nitrates into watersheds which is a threat to human health and the environment. Carbon nanotubes (CNTs) are effective catalysts for reducing nitrate pollutants to useful ammonia products. In collaboration with the Wang group, we are studying the mechanism behind CNT-catalyzed nitrate reduction to ammonia.
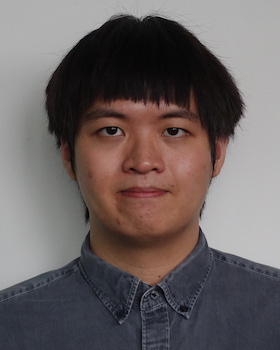
Tianyin (Tim) Qiu
The study of time evolution in conventional theoretical chemistry relies on solving a series of physics motivated equations for specific model systems. For example, classical molecular dynamics is preceded by numerically integrating Newtonian motions, while quantum dynamics mostly focuses on solving the time-dependent Schrödinger equation. Both approaches require one to know the Hamiltonian of the whole model system to forecast incoming events. While such calculations are insightful and predictive, some systems can be too expensive to calculate, or even, in some cases, not possible even with high performance computing.To tackle such limitations, I propose to explore the development of methods to study the evolution of observables without having to solve the evolution of the system over the whole simulation time. The idea is to build propagators of observables from the early time dynamics and to use the resulting propagators to forecast the evolution of observables. Developing that type of method requires two steps: (1) Build a model system which captures the key features of the system from the known observables. (2) Forecast the future evolution from the trained model. While the final goal is to predict the long-time dynamics, as described in the second aim, the first goal is a lot harder, yet a prerequisite for forecasting.
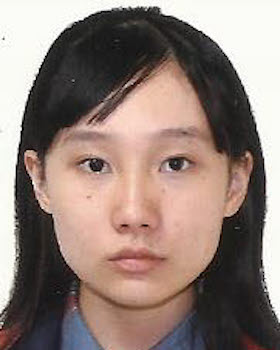
Yuanjun (Sherlock) Shi
Understanding the RNA synthesis of the nsp12 Polymerase of SARS-CoV-2 Using Molecular Dynamics SimulationsThe RNA-dependent RNA polymerase (RdRp, nsp12) of SARS-CoV-2 is responsible for RNA synthesis of viral mRNAs and new RNA genome within the replication-transcription complex (RTC). RdRp is a primary antiviral target that could slow down or inhibit viral RNA synthesis. Remdesivir (RDV) is a 1’-cyano analogue of adenosine that binds at the active site of RdRp. I have carried out molecular dynamics (MD) simulations to study the translocation pause after incorporation of RDV monophosphate into the primer at the third primer position. I also studied the structures and distribution of ground and activated states of the RDV bound to nsp12 RNA polymerase.
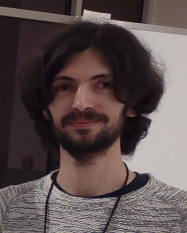
Delmar Azevedo Cabral
Spin-Dependent Electronic StructureOne of the fundamental components of a comprehensive description of chemical systems at the atomic level is the consideration of electronic degrees of freedom. My current research is primarily focused on the development of computational tools and techniques for modeling the electronic structure of chemical systems with spin-dependent components. Specifically, I am interested in exploring the explicit role of electronic spin in better describing photochemical processes, magnetic control of chemical reactivity, and chiral-induced spin-selectivity in large chemical systems with the aim of developing improved materials for catalysis and energy-related applications.
Quantum Computing Algorithms for Chemistry
In addition to these pursuits, my research involves the application and refinement of quantum computer algorithms for solving complex chemical problems. As part of this work, I am simulating the electronic structure of chemical systems with the nuclear electronic orbital description of protons, in collaboration with the Hammes-Schiffer group at Yale.